Big Changes are on the Horizon for EdTech Platforms Courtesy of Big Data. The Question? How will the Industry Leverage Data in 2022 and Beyond
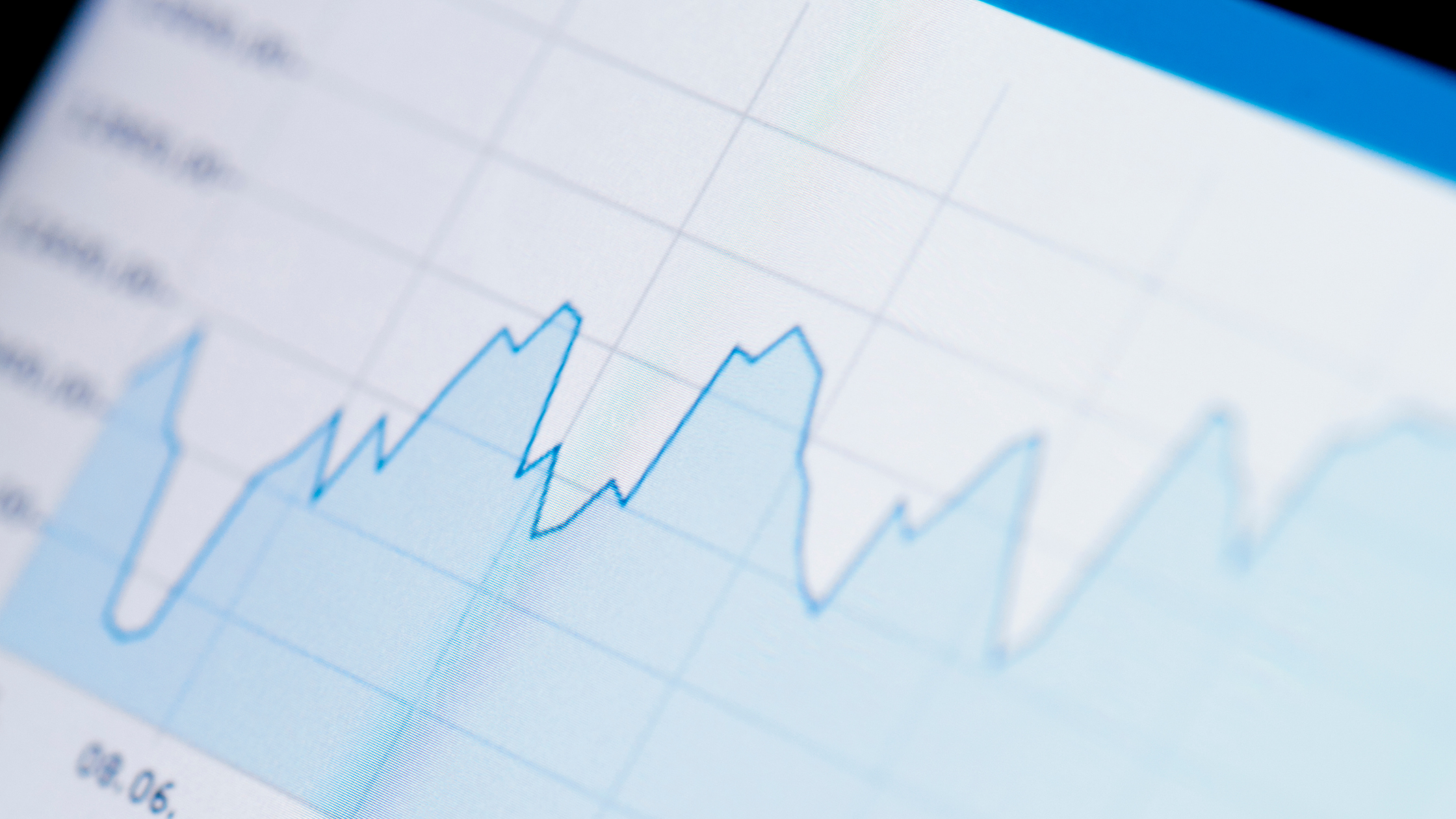
If there is one thing you can say about the pandemic it is that it has transformed entire industries. Take EdTech where the sudden move to virtual learning has provided a flood of data to organizations allowing them to create complete pictures of the individual learner.
The data revolution continues and as a result, today’s EdTech organizations must be secure and data-driven to be competitive and effective. Ultimately data is key to unlocking EdTech solutions.
Think Systems, Inc. hosted a panel discussion bringing together educational technology experts in academia, technical development, and software to discuss ways EdTech organizations can effectively harness big data and use it to drive user experience, engagement, and growth.
Panelists included: Andrew Hampton, Assistant Professor of Psychology at Christian Brothers University, and Chair of the IEEE Standards Association Working Group for Adaptive Instructional Systems; Phil Horowitz, Chief Architect at JBS Custom Software Solutions; and Russ LeWinter, PMP, CSPO, Head of EdTech/GovTech Ideation at Amazon Web Services.
Q: What is the significance of big data in the educational sector?
Hampton: That’s a big question and the simplest answer is that we don’t know yet. Most of the data we’re trying to leverage came about because it was easier to put assessments, quizzes, and exercises online. When you do that, it’s easy to gather data.
We’re getting to the point now we have so much information about students and learners of every age and sector across the world and that data has so much raw potential. We have ideas but putting those into practice is a much bigger challenge.
Q: What is going to be the breakout area for improving education?
Hampton: Where we can go in the future, is test new theories that we haven’t had any capacity to test in the past.
Getting rid of standardized testing is completely plausible. Every time you have an interaction with a software program or educational technology, that is an assessment. Instead of one giant three-hour assessment, we have a hundred assessments over the course of a day or a classroom session, whatever the level of granularity and analysis you want to have. We could create a much more comprehensive picture of an individual learner.
Q: We often think about EdTech as K-12 and higher education, but corporations have learning management systems and higher learning enterprises. Do you see those coming together or sharing technologies in anyway?
LeWinter: The learning standards and data interoperability are still being formed. It’s one area we’re looking at ways to pull different data from different systems. We’re seeing this in the corporate space.
Another trend we’re hearing is around upscaling, how can we take data that was gathered in high school and college and help individuals get the next job or identify different areas they can work on.
Unfortunately, in EdTech, there’s no global repository for data that others can use to leverage and analyze in a meaningful way, but I predict that we will get there eventually. It’s still early in breaking down those silos.
Q: What are the challenges of collecting so much data and how can systems put it to use?
Horowitz: From a challenge perspective there are three key areas that most systems in production today are having problems with.
In my experience, those three things are:
- Volume and velocity. Most systems can’t handle volume and velocity of data that we want to see in modern big data systems. How do we make systems that can handle the volume of data to do analytics and gather insights?
- Systems integration. There’s been a deluge of all sorts of multimedia content, video, audio, online meetings, etc. How do we get these older legacy systems talking to newer systems or other kinds of systems? How do we export data that talks to each other?
- Outdated systems. Many of these systems have been in production prior to rise of analytics in machine learning and predictive analytics. Many of AI and ML features are not truly integrated in the system as whole and are simply bolted on. It is difficult to get these systems updated to do what we want moving forward.
LeWinter: We tend to take that crawl, walk, run approach, because first, you need to get that data in order. We’ll work with customers in the “crawl phase,” which is just investigating what data they have, what data we can use, and what data is important to them.
Then we move onto the “walk phase.” How do you get things like video put into a text base to improve search results when you’re looking for a video asset? AWS offers services I call more in the middle versus advanced machine learning technologies that our customers implement immediately. For example, Amazon Personalize, uses predictive analytics similar to the suggested items on your Amazon Prime page. It uses a lot of data that we have on the backend, which would be considered the “walk phase.”
Then we get into the “run phase” using advanced machine learning where you start building custom models on top of that data and have the ability to accomplish really powerful things.
Q: How is the data unlocking education technology solutions?
Hampton: Not by itself, the data that we’re creating is largely speculative. How that data starts changing things is going to inform how we’re going to handle that data. We’re just beginning to wander into the wild west, where the data resides, and what we can do with it.
Q: A lot of EdTech platforms are built in multiple silos or legacy platforms. How do you make these tools accessible?
LeWinter: A lot of these services have prebaked models built in, so you don’t have to start from scratch. For example, Amazon’s Quick Site allows you to put data in and start building visualizations. You don’t have to sign long-term contracts, which allows the democratization of AI and ML data and a solution to quickly and easily help our EdTech’s.
Q: How do you firewall from bias in data? What’re the challenges?
Hampton: Awareness is certainly the first step. We have the opportunity to democratize education worldwide or we can replicate systems we already have in place and make it better.
AI also has the potential to institutionalize biases more than they already are. If you take race out of consideration with a body of students, we have what’s called the reconstruction problem, where AI will continue to recreate and be race blind. We are trying to put meaningful barriers in place.
Horowitz: This conversation is very vital. I can see this becoming the biggest topic in EdTech as data continues to grow. We need to be aware of the problem and mitigate bias as much as we can.
A lot of data sets are too large to look at by eye and see the bias. Tools have been built with algorithms to determine what kinds of biases your data set is exhibiting, and these tools will help us tackle these problems.
Q: We’re at a time when technology is ahead of established codes and best practices. How do we move forward in EdTech from a policy perspective?
Hampton: We need to maintain our researcher hats because we are in an unregulated domain where data is recognized as powerful. We have these tools that have the human capacity to understand, and we must approach problems mindfully. Efficiency isn’t justice and not always the best solution. Moving forward we need to keep those principles in mind.