Using AI to Move Beyond Individual Mastery
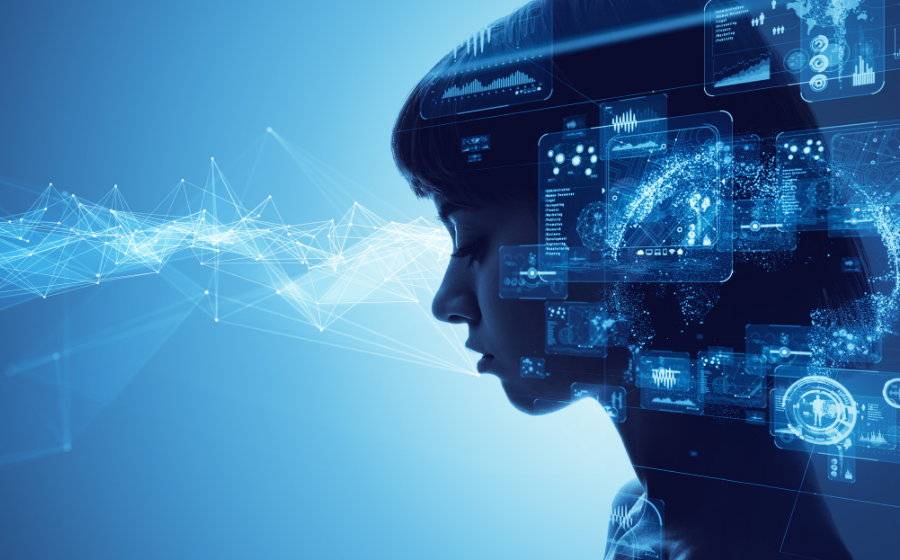
Few spaces were rocked harder by the COVID-19 pandemic than the field of education. From adapting to a full remote learning model on the fly to continuing disagreement over the safety of returning to in-person learning, it’s been a truly disruptive year for educators.
For those reasons, there has been perhaps an even stronger focus than usual on technological advancements in education, and especially on the role that artificial intelligence (AI) might play going forward.
At a recent EdTech Maryland Meetup we were brought up to date on the topic by a comprehensive presentation from Andrew J. Hampton, PhD, a research scientist and Assistant Professor at the University of Memphis; and Philip Horwitz, Chief Architect at JBS Custom Software Solutions.
Regarding education in general, Andrew led off with this provocative statement: “COVID didn’t break everything; it showed us where the cracks had always been.” In education, perhaps the most significant of those cracks involves the traditional one-to-many classroom model, unchanged for more than a century. The teacher presents the same information to everyone in the class simultaneously.
The obvious problem with the traditional model is that students don’t learn at the same pace, so classroom instruction tends to either leave some students behind or gravitate to the lowest common denominator, limited to the pace of the slowest learners. Recent evolution in classroom design has allowed for smaller group settings to address this to some degree, but not to an extent that can be considered individualized learning.
Enter computer-based instruction, wherein a student interacts individually with a system. In theory, this is more efficient in allowing personalized learning, mastering one task and then moving on to the next. While this represents progress, it’s essentially a one-way street; the system understands only that correct responses have or have not been entered.
And that’s where AI can begin to make a difference, in the form of adaptive instructional systems. This model also features one-to-one interaction between student and system, but with more individualized assessments of the strengths, weaknesses and needs of each student. Properly designed, AI can go beyond simply checking the boxes of right or wrong answers and begin to understand which concepts a student has mastered and which they still might be struggling with.
As with any technology in its infancy, there are hurdles to be overcome, primary among them a lack of standards, especially around interoperability of disparate systems and a full understanding of ethical implications (Andrew chairs an IEEE standards group addressing these and other issues).
In a detailed look under the hood at both the opportunities and challenges in undertakings like this, Andrew cited an Office of Naval Research Grant/STEM Challenge around education in electrical engineering. In an unusual twist, the ONR chose five entries of the many submitted and charged the entrants with combining them into a single project, quite the challenge.
The solution involved breaking the five entries into a total of 120 individual components, which were then combined into a single interface. The result allows learning in this case to move beyond a step-adaptive approach (student answers question incorrectly; gets another question on the same topic). In fact, the model allows both a narrower and wider lens, with feedback at multiple levels ranging from an even closer look (micro-adaptive) to the proverbial 10,000-foot view of the topic (meta-adaptive).
All student data is saved in a learning record store, where machine learning can step in to parse that data and calculate performance, generate possibilities and even recommend the next three steps for improved learning.
One natural concern as machine learning becomes more prevalent is over the continuing role of the instructor. Andrew pointed out that these models are designed to work alongside human instructors, not to cut them out of the picture.
Phil then noted some additional detail as to how exactly this model becomes reality, including a look at AWS services that both comprehend and generate natural language to improve communication with the learner. Use of the AWS platform lowers the barrier to entry in such endeavors with automatic scaling, automated workflows and a secure, encrypted platform that integrates easily with other AWS services.
At this early stage there are perhaps more questions than answers around the applications of AI in education, especially in the technical and ethical hurdles mentioned above. As Andrew notes, “Innovation is wasted without integration,” and the need for disparate systems to work in concert will be paramount as well.
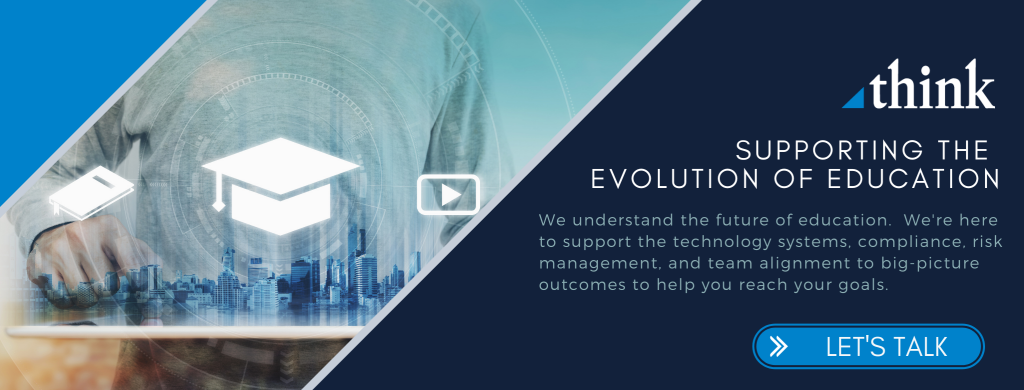